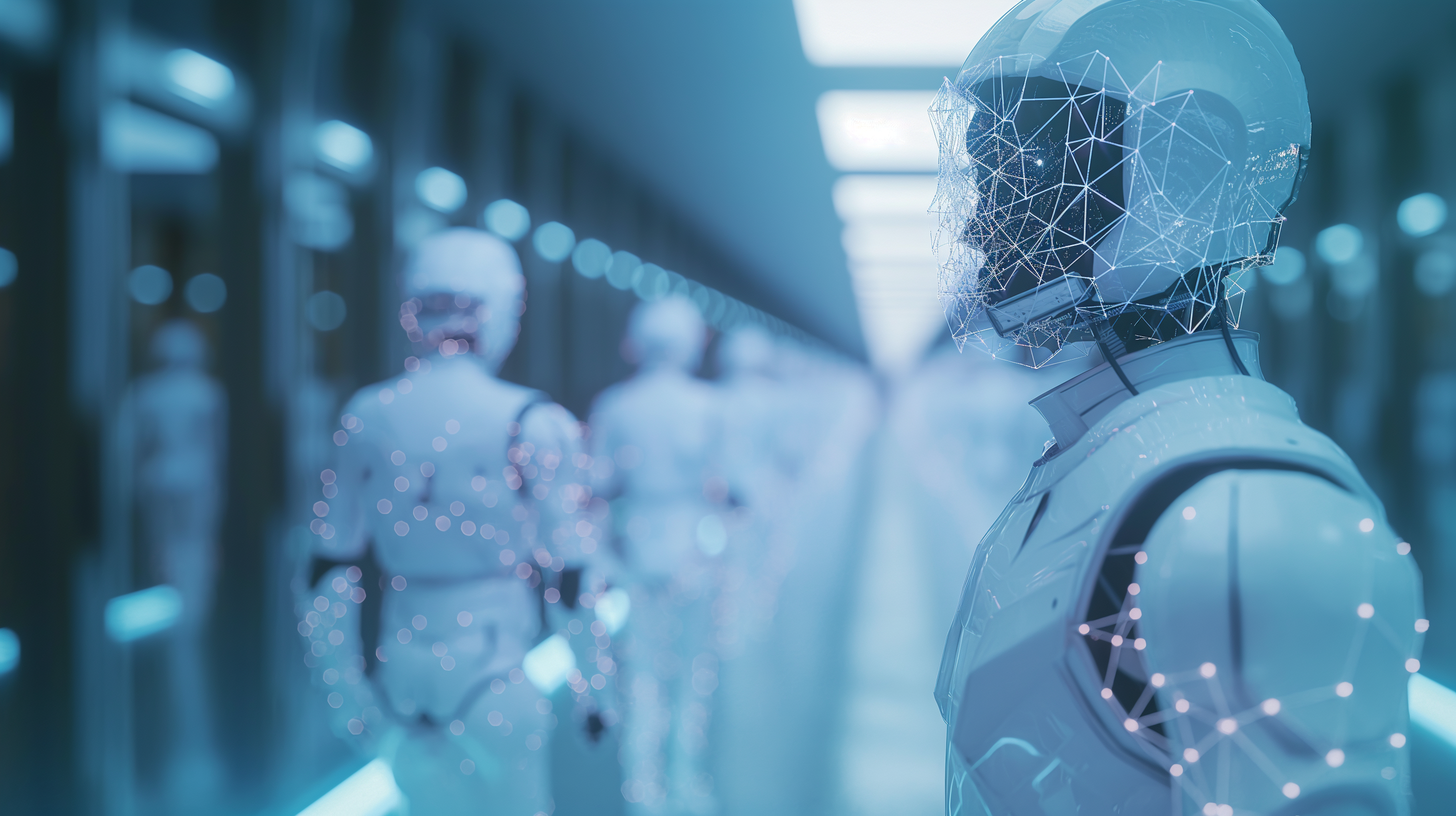
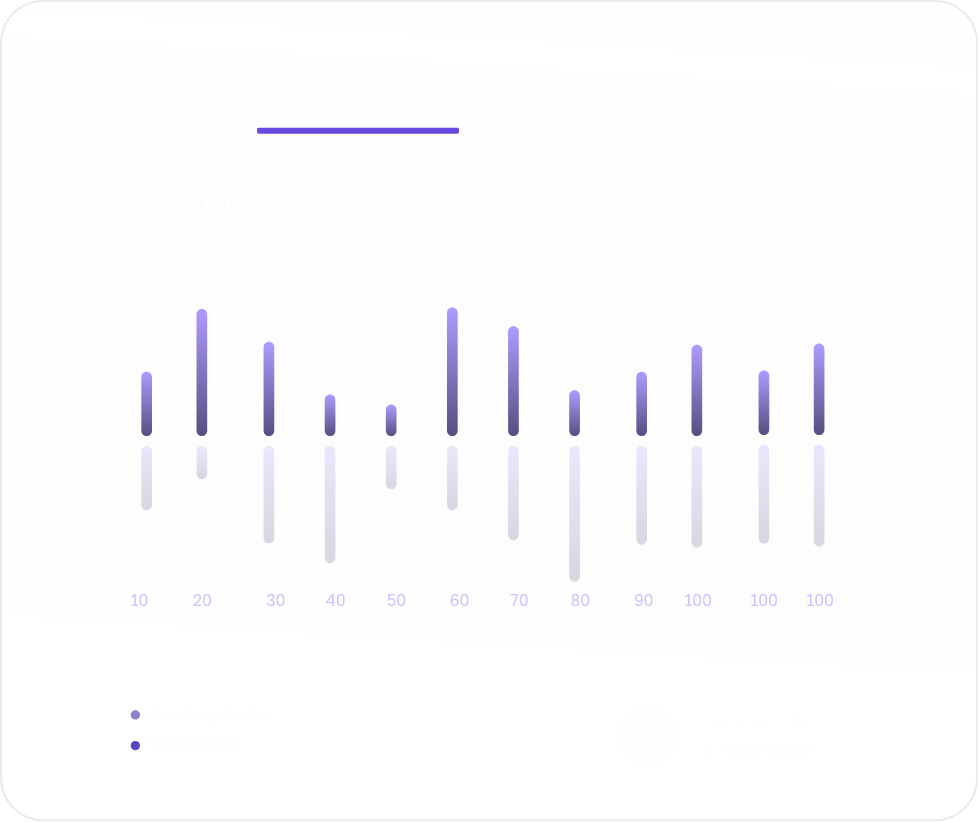
Unleashing the Potential of Multi-Agent AI Models
Artificial Intelligence (AI) continues to revolutionize various industries, offering innovative solutions and transforming business operations. In this article, we delve into the realm of Multi-Agent AI Models and their integration into middle-sized companies' workflows. We explore how Actionable AI, driven by these advanced models, can enhance decision-making, efficiency, and overall business performance. Drawing insights from recent advancements and other reliable sources, we provide a comprehensive guide for middle-sized companies to harness the power of Multi-Agent AI Models.
Introduction to Multi-Agent AI Models
Definition and Overview
Multi-Agent AI Models represent a significant advancement in the field of artificial intelligence. Unlike traditional AI systems, which often rely on a singular decision-making process, Multi-Agent AI involves multiple autonomous agents working together to achieve complex objectives. Each agent operates with a degree of independence, yet they collaborate and communicate to solve problems more efficiently. This distributed approach allows for flexibility, scalability, and robustness in handling a wide range of tasks.
Evolution of AI Models
The journey from simple rule-based systems to sophisticated Multi-Agent AI Models has been transformative. Initially, AI systems were limited to executing predefined rules and lacked the ability to adapt to dynamic environments. However, advancements in machine learning, particularly reinforcement learning and deep learning, have paved the way for more adaptive and intelligent systems. Multi-Agent AI Models capitalize on these advancements, offering a collective intelligence that can surpass individual capabilities.
Understanding Actionable AI
Concept of Actionable AI
Actionable AI refers to the subset of artificial intelligence that not only processes data but also translates insights into concrete actions. It bridges the gap between data analysis and decision-making, enabling businesses to act swiftly and effectively based on AI-driven recommendations. Unlike traditional AI systems that may require human intervention to interpret results, Actionable AI can autonomously execute decisions, thereby streamlining processes and reducing latency.
Difference Between Traditional and Actionable AI
The key difference between traditional AI and Actionable AI lies in their operational scope. Traditional AI focuses primarily on data processing and analysis, providing insights and predictions. However, these systems often rely on human decision-makers to interpret and act upon the results. In contrast, Actionable AI integrates the decision-making process, automating actions based on real-time data. This capability makes it particularly valuable in dynamic and fast-paced business environments where quick responses are crucial.
The Role of Multi-Agent AI Models in Actionable AI
How Multi-Agent Systems Operate
Multi-Agent AI Models operate by distributing tasks among various agents, each with specific roles and responsibilities. These agents can be specialized for particular functions, such as data collection, analysis, or decision-making. The collective intelligence of the system emerges from the interactions and communications among agents, enabling the system to tackle complex problems that may be beyond the scope of a single agent.
Synergy Between Agents
The synergy between agents in a Multi-Agent AI system is pivotal. Agents can share knowledge, learn from each other’s experiences, and adapt to new situations. This cooperative behavior enhances the system’s overall efficiency and effectiveness. For instance, in a business scenario, one agent might monitor market trends while another optimizes inventory levels, and a third handles customer service queries. Together, they form a cohesive unit that ensures smooth operations and maximizes business outcomes.
Use Cases in Middle-Sized Companies
Middle-sized companies stand to benefit immensely from the implementation of Multi-Agent AI Models. These systems can streamline operations, optimize resource allocation, and improve customer engagement. For example, a Multi-Agent AI system can automate supply chain management, predict demand fluctuations, and adjust procurement strategies accordingly. In customer service, agents can handle routine inquiries, allowing human representatives to focus on more complex issues. Such applications not only enhance efficiency but also contribute to a better customer experience.
Contributions to Multi-Agent AI Models
AI Frameworks
The development of Multi-Agent AI Models has been greatly supported by advancements in AI frameworks and platforms. These frameworks provide the necessary infrastructure for training and deploying complex AI systems, facilitating the creation of robust and scalable solutions. The comprehensive suite of tools available in these frameworks supports various AI workflows, from data preparation to model training and inference, making them ideal for businesses looking to integrate AI into their operations.
Notable Projects and Innovations
Beyond frameworks, there have been numerous innovations in AI hardware and software that have significantly accelerated the computational capabilities required for training deep learning models. Notable projects in fields such as autonomous systems demonstrate the use of Multi-Agent AI to coordinate the actions of multiple autonomous agents in a shared environment. This technology not only enhances safety but also optimizes operations in various domains, such as logistics and transportation.
Integration of Actionable AI in Business Workflow
Initial Steps for Integration
Integrating Actionable AI into a business workflow begins with identifying the key areas where AI can add value. This process involves conducting a thorough analysis of existing processes, identifying pain points, and setting clear objectives for AI implementation. Middle-sized companies should start with pilot projects that address specific challenges, allowing them to test the waters and assess the benefits before scaling up.
Tools and Technologies
The choice of tools and technologies plays a critical role in the successful integration of Actionable AI. Companies should consider platforms that offer flexibility, scalability, and ease of use. Various cloud-based platforms offer AI services that can be tailored to specific business needs, providing a solid foundation for developing and deploying Multi-Agent AI Models. These services include data storage, model training, and deployment, making it easier for businesses to leverage AI technology.
Key Considerations and Challenges
While the benefits of Actionable AI are substantial, companies must also navigate several challenges. Data privacy and security are paramount, particularly when dealing with sensitive customer information. Ethical considerations, such as ensuring unbiased decision-making and maintaining transparency, are also crucial. Moreover, businesses must invest in upskilling their workforce to effectively manage and operate AI systems. Addressing these challenges proactively can pave the way for a smooth and successful AI integration.
Advantages of Multi-Agent AI Models for Middle-Sized Companies
Cost Efficiency
One of the primary advantages of Multi-Agent AI Models is cost efficiency. By automating repetitive and time-consuming tasks, these systems reduce the need for manual intervention, allowing companies to allocate resources more effectively. This automation can lead to significant cost savings, particularly in areas such as customer service, where AI agents can handle routine queries without human assistance.
Scalability
Multi-Agent AI Models offer unparalleled scalability. As businesses grow, these systems can easily accommodate increased workloads without compromising performance. The modular nature of Multi-Agent AI allows companies to add or modify agents as needed, ensuring that the system can adapt to changing business requirements. This scalability is particularly beneficial for middle-sized companies looking to expand their operations.
Enhanced Decision-Making
By leveraging the collective intelligence of multiple agents, Multi-Agent AI Models can enhance decision-making processes. These systems can analyze vast amounts of data from various sources, identify patterns, and provide actionable insights. For example, in marketing, AI agents can analyze customer behavior, predict trends, and optimize campaigns for maximum impact. This data-driven approach ensures that decisions are based on accurate and up-to-date information, leading to better outcomes.
Case Studies: Successful Implementations
Real-World Examples
Several middle-sized companies have successfully integrated Multi-Agent AI Models into their workflows, reaping significant benefits. For instance, a retail company implemented a Multi-Agent AI system to manage inventory and optimize supply chain operations. The system not only streamlined the procurement process but also significantly reduced costs and improved customer satisfaction. Another example involves a logistics firm that utilized Multi-Agent AI to enhance route planning and delivery efficiency, resulting in faster delivery times and reduced operational expenses.
Lessons Learned
From these case studies, it’s evident that a well-implemented Multi-Agent AI system can yield substantial benefits. However, the success of such implementations depends on careful planning, continuous monitoring, and the willingness to adapt to new challenges. Companies should start small, gradually scaling up their AI initiatives as they gain experience and confidence in the technology. It’s also crucial to maintain a clear focus on business objectives and ensure that AI solutions align with the company’s strategic goals.
Actionable AI in Various Business Domains
Customer Service
In the realm of customer service, Actionable AI powered by Multi-Agent systems can transform how companies interact with customers. AI agents can handle routine inquiries, provide personalized recommendations, and resolve common issues without human intervention. This not only enhances the customer experience but also frees up human representatives to focus on more complex and sensitive matters. The result is a more efficient and responsive customer service operation that can handle a higher volume of interactions.
Supply Chain Management
Multi-Agent AI Models can optimize supply chain management by providing real-time insights into inventory levels, demand forecasts, and supplier performance. AI agents can predict potential disruptions, recommend optimal inventory levels, and automate procurement processes. This proactive approach helps companies maintain a smooth supply chain, reduce costs, and prevent stockouts or overstock situations. By leveraging AI, companies can achieve greater visibility and control over their supply chain operations.
Marketing and Sales
In marketing and sales, Actionable AI can analyze customer data to identify trends, preferences, and purchasing behaviors. AI agents can segment customers, personalize marketing campaigns, and predict the success of various strategies. This targeted approach ensures that marketing efforts are more effective, reaching the right audience with the right message. Additionally, AI-driven sales optimization can streamline the sales process, from lead generation to conversion, resulting in higher revenue and customer satisfaction.
Steps to Implement Multi-Agent AI Models
Developing a Strategic Plan
The first step in implementing Multi-Agent AI Models is to develop a strategic plan that outlines the company’s objectives, resources, and timelines. This plan should identify the specific areas where AI can add value and define the metrics for success. It’s essential to involve key stakeholders from the outset to ensure alignment with the company’s overall strategy and secure the necessary support for the project.
Choosing the Right AI Solutions
Selecting the right AI solutions is crucial for the success of the implementation. Companies should evaluate various AI platforms and frameworks to determine the best fit for their needs. Factors to consider include ease of use, scalability, and the availability of pre-built models and tools. It’s also important to assess the level of support and training provided by the vendor to ensure a smooth deployment.
Training and Development
To fully leverage the potential of Multi-Agent AI Models, companies must invest in training and development. This includes upskilling existing employees and hiring new talent with expertise in AI and machine learning. Providing ongoing training and support will ensure that the workforce is equipped to manage and optimize AI systems effectively. Additionally, fostering a culture of innovation and continuous learning will encourage employees to embrace new technologies and drive the company’s AI initiatives forward.
Ethical Considerations and Compliance
Data Privacy
Data privacy is a critical concern when implementing Multi-Agent AI Models. Companies must ensure that they comply with relevant data protection regulations and maintain transparency with customers about how their data is used. Implementing robust security measures and anonymizing data where possible can help mitigate privacy risks and build trust with customers.
Ethical AI Usage
Beyond compliance, companies must also consider the ethical implications of AI usage. This includes ensuring that AI systems are unbiased, transparent, and accountable. Companies should establish guidelines for ethical AI practices and regularly audit their AI systems to identify and address any issues. By prioritizing ethical AI usage, companies can not only avoid potential legal and reputational risks but also demonstrate their commitment to responsible innovation.
Future of Multi-Agent AI Models and Actionable AI
Emerging Trends
The future of Multi-Agent AI Models and Actionable AI is promising, with several emerging trends set to shape the industry. One such trend is the increasing adoption of AI in decision-making processes, with more companies leveraging AI to automate and optimize business operations. Another trend is the growing emphasis on explainable AI, which seeks to make AI systems more transparent and understandable to users.
Predictions and Forecasts
As technology continues to evolve, we can expect to see more sophisticated and capable Multi-Agent AI Models. These systems will likely become more integrated into various business functions, from customer service to supply chain management. Additionally, advancements in AI hardware and software will further enhance the performance and scalability of these models. Companies that invest in AI today will be well-positioned to capitalize on these advancements and stay ahead of the competition.
Conclusion
The integration of Multi-Agent AI Models and Actionable AI offers immense potential for companies. By automating processes, optimizing operations, and enhancing decision-making, these technologies can drive significant business growth and competitiveness. As companies continue to explore and adopt these innovations, they must also consider the ethical and practical implications, ensuring that AI is used responsibly and transparently. The future of AI is promising, and businesses that embrace these technologies today will be well-equipped to navigate the evolving landscape of digital transformation.
FAQs
What are Multi-Agent AI Models?
Multi-Agent AI Models involve multiple autonomous agents working together to achieve complex objectives. Each agent operates independently yet collaborates with others, allowing the system to tackle a wide range of tasks more efficiently.
How can middle-sized companies benefit from Multi-Agent AI Models?
Middle-sized companies can streamline operations, optimize resource allocation, and enhance customer engagement. These systems offer cost efficiency, scalability, and improved decision-making capabilities.
What are the challenges of integrating Actionable AI?
Key challenges include data privacy and security, ethical considerations, and the need for upskilling the workforce. Addressing these challenges proactively is crucial for a smooth and successful integration.
How do Multi-Agent AI Models improve decision-making?
By leveraging the collective intelligence of multiple agents, these models can analyze vast amounts of data, identify patterns, and provide actionable insights. This data-driven approach leads to better and more informed decisions.
What are some use cases of Multi-Agent AI in business?
Use cases include customer service, supply chain management, marketing, and sales. AI agents can handle routine tasks, optimize processes, and personalize customer interactions.
What is the future of Multi-Agent AI Models and Actionable AI?
The future is bright, with emerging trends such as explainable AI and increasing AI integration in decision-making. Continued advancements in technology will enhance the capabilities and applications of these models.